11 min read
Responsible AI in Financial Crime Compliance: A Path Towards Effective and Ethical AI-Assisted Anti-Money Laundering and Counter-Terrorist Financing
Leandro Loss, PhD
:
December 19, 2024
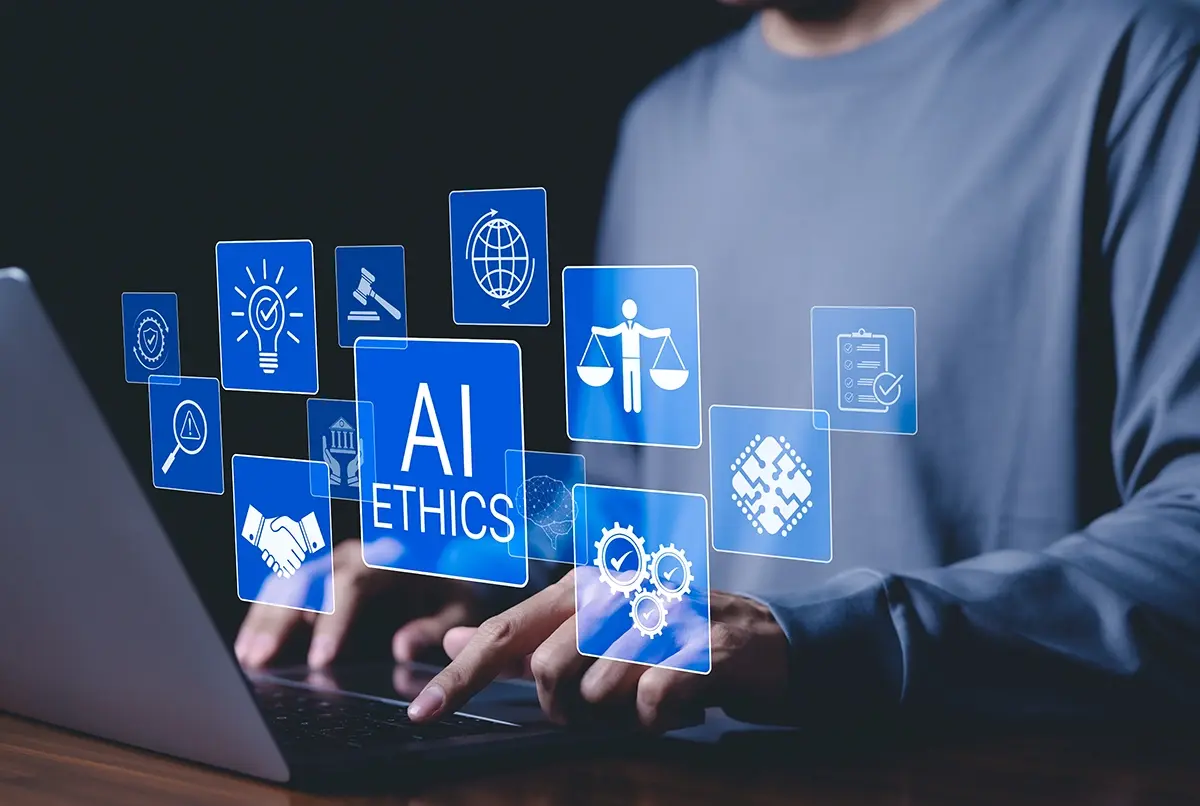
Leandro Loss, PhD is the
Principal Data Scientist/Head of AI R&D
at AML RightSource
Introduction
The rise of Artificial Intelligence (AI) and, more recently, Generative AI (GenAI) has been transforming industries worldwide, offering affordable and innovative solutions to complex problems historically solved only by a scarce, specialized workforce. AI has shown immense potential in combating financial crimes, particularly in Anti-Money Laundering (AML) and Counter-Terrorist Financing (CTF) efforts. However, as with any tool, the application of AI in AML/CTF must be approached with responsibility and ethical considerations.
Responsible AI refers to the design and development of traditional or generative artificial intelligence systems in a manner that aligns with an organization’s ethical principles and values. In this article, I propose a comprehensive framework for implementing AI systems for financial crime compliance and other highly regulated industries with high-quality standards. This framework addresses all practical aspects of Responsible AI in a six-fold solution that can be referred to, for simplicity, by the acronym E-T-H-I-C-S:
- Enhancing: Contributing positively to society, its institutions, and above all, its people.
- Transparent: Providing clear explanations about how decisions are made.
- Human-Centered: Prioritizing human values, needs, and experiences.
- Imputable: Allowing for monitoring, auditing, and accountability of their actions and decisions.
- Credible: Being truthful and avoiding biases to ensure equitable outcomes for all and every case and scenario.
- Secure: Protecting personally identifiable information and other user data, ensuring privacy and confidentiality.
Ultimately, Responsible AI aims to align technology with human values and rights. To this end, the ETHICS framework was created to provide AI technologists and stakeholders with a concrete foundation for understanding and deploying Responsible AI. The remainder of this article delves into the role of Responsible AI in enhancing AML/CTF processes, exploring its benefits and challenges, and the importance of controlled practices to safeguard the industry against ETHICS violations.
Understanding AML/CTF and the Role of AI
AML and CTF are policies and measures that aim to prevent criminals and terrorists from using the financial system. Financial institutions are required to implement stringent AML/CTF measures to detect and report suspicious activities. Traditionally, these measures relied heavily on specialized, manual processes and rule-based systems, often resulting in high costs, inefficiencies, inconsistencies, and high false-positive/false-negative rates. Additionally, the breadth of services and the global nature of the modern financial system require a trained workforce proficient in many products, technologies, and foreign languages.
Alternatively, AI and its more recent algorithmic strategy, AI, have emerged as a game-changer in this domain, offering human-level abilities that range from basic text processing for translations and summarizations to advanced pattern recognition and reasoning that can be employed in entity resolution, adverse media monitoring, risk assessment, and several other problems. Generally speaking, ingesting vast amounts of data during training and the proper guidance during their operation allows AI systems to perform investigative and compliance tasks faster and more accurately than human beings, becoming a powerful assistant to domain experts, analysts, and compliance officers alike.
Benefits of AI in Financial Crime Compliance
Financial institutions that establish and foment symbiotic relationships between their workers and AI have reported significantly reduced investigation times and compliance costs, resulting in false positives/false negative rates. Not surprisingly, they also observed a lower attrition rate among their specialized workforce, who now find themselves in more rewarding careers where they can focus on finding bad players and illegal activity rather than clearing false alerts created by legacy, rule-based systems. The undeniable and quantifiable outcome these organizations achieve is a more effective and economical compliance operation with stronger financial crime deterrents than traditional methods. Beyond the successes of financial institutions, this represents a significant victory for society and a setback for terrorists and criminals.
Here are some key benefits that financial institutions have discovered using AI:
- Enhanced Detection and Efficiency: AI can quickly process and analyze large datasets, accounting for poor data quality and identifying unusual patterns and transactions that might indicate money laundering and terrorist financing. Simultaneously, it can compare past cases and historical decisions, consult the latest regulations and guidelines, and follow reporting protocols. This increases alerts' efficiency, quality, and consistency, allowing investigators to concentrate on more critical validations and decisions while permitting financial institutions to respond promptly to suspicious activities.
- Reduction of False Positives and False Negatives: Traditional AML systems often produce numerous false positives and unmeasured false negatives, resulting in excessive investigative work, inefficient resource use, flawed compliance processes, regulatory scrutiny, and potential financial and reputational penalties. AI can enhance efficiency by learning and adapting to new patterns rather than relying solely on rules, thereby improving the accuracy and identification of valuable alerts. Moreover, AI can detect unexpected behaviors and anomalies, revealing otherwise overlooked activities.
- Multilingual Capabilities: Modern AI systems have advanced natural language processing (NLP) to accomplish tasks in hundreds of languages. This enhances the detection of suspicious activities across different regions and linguistic contexts. Such capabilities allow investigators to automatically access and produce information in languages and quality levels previously unavailable, improving cross-border collaboration and ensuring comprehensive coverage of global financial activities. This multilingual proficiency helps us understand nuanced language differences and cultural contexts, leading to more accurate and effective AML efforts.
- Speed and Scalability: When integrated with a robust and modern infrastructure, AI systems can quickly scale to handle increasing volumes of data and transactions. This scalability is crucial for global financial institutions that face diverse and dynamic AML challenges, allowing them to manage peak loads and expand datasets without compromising performance and consistency. The speed and scalability of AI enable real-time monitoring and quick response to emerging threats, ensuring institutions remain agile and proactive in their AML strategies.
- Adaptation and Continuous Learning: AI can continuously learn from new data and scenarios, allowing it to adapt to evolving money laundering and terrorist financing techniques and regulatory requirements. This continuous learning capability ensures that AI models remain relevant and effective in the face of changing criminal strategies and regulatory landscapes. By staying current with the latest trends and patterns, AI systems can provide insights that drive strategic decision-making and enhance overall compliance efficacy.
- Lower Attrition Rate Among Analysts: By reducing repetitive tasks and false positives, AI allows analysts to focus on more complex and meaningful work. This shift in workload increases job satisfaction and reduces turnover rates as analysts engage in tasks that utilize their expertise and analytical skills. Reducing ordinary tasks also decreases burnout, fostering a more motivated and stable workforce. Additionally, AI handling routine operations gives analysts more professional development and innovation opportunities, further contributing to a positive work environment.
Challenges and Practical Ethical Considerations
While AI brings substantial and quantifiable benefits to AML/CTF, its design and deployment must be handled responsibly to mitigate potential social and compliance risks. Such risks often carry a possible detrimental impact that is perceived as far surpassing AI’s benefits, justifiably creating resistance among the high ranks of financial institutions. Here are some key challenges and ethical considerations highlighted by organizations:
- Unreliable and Inconsistent Quality: The data and algorithms used in AI systems can sometimes be of questionable quality, leading to inaccurate results and high rates of false positives and negatives. Obscure evaluation practices further complicate the ability to assess the system's effectiveness, while challenges in reproducibility hinder consistent performance across different scenarios. These issues result in extra work for analysts who must manually verify and rectify poor results, reducing overall efficiency. Data integrity, transparency in algorithm design, clear evaluation criteria, and reproducibility are crucial to maintaining the system's credibility and reliability.
- Lack of Transparency and Explainability: AI models, particularly large and complex ones based on deep learning and large language models, can be opaque, making it difficult to understand how decisions are made. The lack of transparency and inconsistent results pose challenges for accountability and regulatory compliance, as stakeholders may find it hard to trust systems they cannot comprehend. Developing transparent and explainable AI is essential for fostering trust and understanding among stakeholders. In addition, deploying interpretation mechanisms could mitigate the distrust of traditional “black box” models, making AI systems more user-friendly.
- Unwieldy Human Oversight and Accountability: While AI can automate many aspects of AML/CTF, human oversight remains essential. In addition to ensuring that AI systems have native monitoring/auditing features, financial institutions find it difficult to establish clear accountability frameworks and ensure that critical AI-driven decisions are subject to human review and intervention when necessary. This would involve defining roles and responsibilities for monitoring AI outputs, identifying risk impacts, investigating potential anomalies, and performing periodic re-evaluations against benchmarks and historical results. In other words, financial institutions would ensure that humans are ultimately responsible for all final decisions. Human oversight also helps address ethical considerations, providing a safety net to catch errors or biases that AI might miss. The lack of accountability mechanisms and human control makes training the staff in oversight roles impossible, leading to ineffective human-AI collaboration.
- Ad-Hoc Regulatory Compliance: Financial institutions must navigate a complex regulatory landscape that varies across jurisdictions and financial modalities. AI systems must be designed to comply with these regulations while maintaining flexibility to adapt to changes. This requires staying informed about regulatory updates and ensuring that AI models are easily auditable and capable of demonstrating compliance. Collaboration with legal experts and regulators during the design and deployment phases is also necessary to help align AI systems with current and future regulatory expectations. Closed access to utilized sources and their refresh frequency hinder an organization’s ability to assess compliance risk and prevent “blind spots.”
- Bias and Unfairness: AI systems can inadvertently perpetuate or exacerbate biases present in training data, leading to unfair treatment of specific individuals, languages, countries, and services. This can result in discriminatory practices, such as disproportionately flagging transactions from particular demographics or monetary practices, which consequently promote high rates of false positives and false negatives. Ensuring AI models are trained and evaluated on diverse and representative datasets is crucial to maintaining fairness. Manual audits and bias detection efforts are costly and should be facilitated by AI systems.
- Weak Security and Data Privacy Controls: The use of AI in AML/CTF involves processing sensitive financial data, among many other open and proprietary sources. Ensuring robust data privacy, security measures, and effective data governance is critical to protect individuals' rights and comply with regulatory standards. This includes implementing strong encryption, access controls, and anonymization techniques to safeguard data. Regular security assessments and updates are necessary to protect organizations against malicious exploitation. However, these measures can be costly, time-intensive, and potentially disruptive to internal operations.
Best Practices for Implementing Responsible AI in AML/CTF
Implementing AI for financial crime compliance requires a balanced approach that harnesses the power of technology while addressing ethical and regulatory challenges. By adhering to best practices, financial institutions can ensure that AI systems are effective, fair, transparent, and aligned with regulatory standards. Here is a short list of critical best practices for Responsible AI implementation in AML/CTF:
- Quantitative Performance Metrics: Quality must be measurable. Period. To ensure responsible AI implementation in AML/CTF and guarantee enhancement of human experience and performance, it's crucial to establish clear quantitative metrics, such as false positives and negatives, precision and recall, or other standard metrics aligning with each task's goals. Monitoring these metrics helps detect blind spots and performance drifts while benchmarking against historical results and industry standards ensures progress, competitiveness, and compliance.
Thresholds and hardcoded rules must be reported. Their selection, whenever possible, must be scientific and aim at maintaining a healthy balance between sensitivity and specificity, reducing missed alerts without neglecting the importance of low false alarm rates. Arbitrarily, privileging one or the other will unavoidably affect quality and the human enhancement factor.
In short, comprehensive reporting and transparency are essential. They enable developers and users to demonstrate the system's reliability using sound statistics from a representative evaluation set. This holistic approach ensures the AI system remains effective, efficient, accurate, transparent, and dependable.
- Explainable Output and Interpretable Models: Responsible AI is based on transparency and explainability. It begins with clear documentation detailing the AI system's design, functionality, data sources, and evaluation methodology, making it understandable to all stakeholders. Stakeholders must be permitted to be engaged throughout design and development to ensure alignment of user requirements and regulations. To this end, AI models and developers must be prepared to explain decisions when necessary. Regularly evaluating these requirements maintains system relevance and trustworthiness.
Invest in developing AI whose output is interpretable and explainable. This enables all people training and operating these systems to understand decision-making, fostering trust and facilitating compliance with regulatory requirements. Prioritizing understanding each output and all automated decisions rather than the mathematical and algorithmic inner workings of large and complex systems often proves less fruitful for non-technical teams. Use strategies like local and surrogate modeling for model simplification and interpretability on such large and complex systems. Additionally, visualization and data neighboring strategies should provide clear intuition for AI-driven decisions.
- Humans in Control of Decision-Making: Incorporating human-centric AI in AML/CTF starts by designing systems that prioritize people’s needs and evolve into symbiotic relationships between AI and humans, who should always feel empowered and in control. Engaging diverse stakeholders, including compliance officers and legal experts, ensures the system addresses varied human needs. Regular process assessments help identify inefficiencies, requirement and regulatory deficiencies, and other unintended consequences, ensuring AI serves human and organizational interests.
Additionally, empowering analysts through training enables them to effectively leverage AI tools, focusing on more engaging aspects of investigations rather than time-demanding and tedious tasks like handling false alerts. AI systems should enhance analysts' workflows and decision-making capabilities by focusing on user-centric design. It should not aim to replace humans but rather to magnify their abilities to enable them to accomplish more in less time. This shift enhances job satisfaction and reduces attrition, as analysts are more engaged in meaningful work, ultimately improving organizational efficiency and effectiveness.
- Continuous Monitoring and Robust Data Governance: Regularly monitoring and auditing AI systems help identify and address biases, deviations, errors, and anomalies. Implementing real-time monitoring tools and conducting periodic reviews maintain system accuracy and reliability. Engaging third-party auditors provides impartial assessments and ensures compliance with industry standards.
Robust data governance frameworks should be in place to ensure data quality, privacy, and security, aligning with regulatory requirements. This involves establishing clear data ownership, access controls, and audit trails, with regular reviews and updates to accommodate technological advancements and regulatory changes.
Active engagement with regulators is crucial to stay informed about evolving AML regulations and ensure AI system compliance. Open communication channels with regulatory bodies are necessary to discuss AI implementation and seek compliance guidance. Participation in industry forums and working groups facilitates the sharing of insights and best practices.
Ultimately, humans must be responsible for the successes and failures of AI systems, retaining final decision-making authority. Financial institutions should, thus, ensure all people involved in designing, implementing, and operating AI systems are fully accountable and aware of their roles. Establishing clear accountability frameworks with defined roles for overseeing AI output, assessing risks, investigating anomalies, and making informed decisions is essential.
- Diverse and Inclusive Datasets: This is possibly one of the most essential practices: prioritize data quality. Utilize datasets from credible sources representing various demographics, languages, and services to train and evaluate AI models. This reduces the risk of bias and ensures fair treatment for all individuals and financial products, enhancing their credibility. Regularly update datasets to reflect changing populations and behaviors and involve diverse teams in data selection and model training. Engaging diverse teams not only brings a variety of perspectives but also enhances the identification of potential biases that might be overlooked. It's crucial to perform bias audits and employ fairness metrics to assess and mitigate bias systematically throughout the AI lifecycle.
Fostering transparency by documenting data sources, selection criteria, and processes for automated decisions allows stakeholders to understand and trust the AI model.
Collaborating with external experts and community stakeholders can further enrich the datasets and model development process. By maintaining a commitment to ethical AI practices and inclusiveness, organizations can build systems that are not only technically robust but also socially responsible.
- Designed for Security: Ensuring security in AI systems involves robust protection of personally identifiable information (PII) and other user data to maintain privacy and confidentiality. Implementing advanced encryption techniques and secure data storage solutions is critical to safeguarding sensitive information from unauthorized access and breaches. Regular security audits and vulnerability assessments help identify potential risks and reinforce protective measures. Establishing strict access controls and authentication protocols ensures that only authorized personnel can access sensitive data.
Additionally, adopting data anonymization techniques, such as data masking, redaction, scrambling, and pseudonymization, can further protect user privacy while allowing for secure data analysis. Compliance with relevant data protection regulations requires regular privacy policy and procedure updates. Employee training on data protection and privacy best practices is vital to fostering a culture of security awareness. Engaging with cybersecurity experts and participating in industry forums can provide valuable insights into emerging threats and innovative security solutions.
Conclusion
Responsible AI in financial crime compliance significantly advances the fight against Anti-Money Laundering and Counter-Terrorist Financing, offering improved detection capabilities, efficiency, and adaptability. However, implementing AI and, especially, GenAI in this domain must be approached with caution and ethical considerations. By prioritizing ETHICS (Enhancing, Transparent, Human-Centered, Imputable, Credible, and Secure), financial institutions can harness the power of AI to build a more efficient, economical, and ethical financial system.
As the landscape of financial crime adapts to the fast pace of AI development, Responsible AI will play a crucial role in safeguarding the integrity of global financial systems while maintaining humans at the center of all activity. Integrating AI into AML/CTF processes presents a profound opportunity and a significant responsibility. The potential for AI to revolutionize financial crime compliance is undeniable, offering enhanced detection capabilities, reduced false positives and negatives, and improved efficiency and scalability. These advancements promise to strengthen the defenses against illicit financial activities and create a more fulfilling work environment for compliance professionals, allowing them to focus on meaningful investigative work.
However, deploying AI in this sensitive domain must be carefully and ethically considered. Ensuring reliability, transparency, and fairness in AI systems is crucial to maintaining trust and compliance with regulatory standards. Financial institutions must remain vigilant in addressing biases, safeguarding data privacy, and ensuring human oversight. By fostering a culture of accountability and continuous learning, organizations can mitigate the risks associated with AI, aligning technology with human values and rights.
Ultimately, the success of AI in AML/CTF hinges on the commitment to Responsible AI practices. By prioritizing diverse and inclusive datasets, engaging multidisciplinary teams, and fostering open collaboration with regulators and stakeholders, the financial sector can harness the full potential of AI while safeguarding against ethical pitfalls. As AI evolves, financial institutions must remain proactive, adaptive, and ethical in their AI strategies, ensuring that technological advancements serve the greater good and contribute positively to society. Through the design and deployment of ETHICS-based Responsible AI systems, the industry can achieve a future where financial systems are more efficient, secure, equitable, and transparent for all.