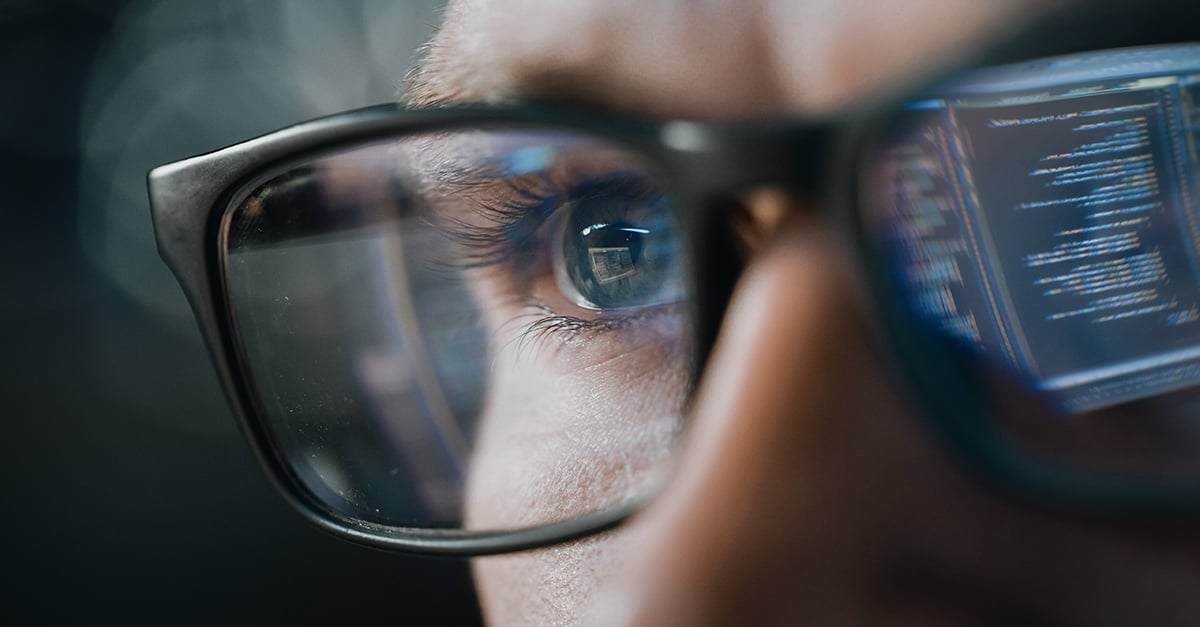
In our latest podcast, Leandro Loss, Principal Data Scientist, and Kevin Lee, Director, Financial Crime Technology, delve into the trustworthiness of AI, why it's often viewed as an enigmatic ‘black box,’ and why conventional models are falling short in the battle against financial crime.
Here’s a recap of what they had to say...
Can We Trust AI?
AI has developed rapidly over the last few years, and there is a lot of discussion about whether we can trust it and the potential risk it poses to society.
Talking about AI in human terms is natural – we look for intentionality everywhere; it's an ingrained instinct.
But AI is a machine. It's an algorithm based on mathematical probabilities. These systems take input and produce output. You cannot apply the concept of trust, an inherently human emotion, to a machine.
Instead, let's ask the people who build and train AI systems if they're trustworthy.
Can we trust them to avoid bias? Can we trust them to develop a system that communicates uncertainty? Can we trust the collection and use of data to train these models?
“We're not talking about the human side of trusting. We're talking about trusting the results of it.” – Leandro Loss.
Ultimately, AI relies on data for decision-making, and any model's accuracy and trustworthiness will depend on the quality of data and whether it is well-programmed.
It's also important to note that AI systems can make mistakes, just like humans, but we can and should trust the results with adequate safeguards that address bias and uncertainty.
Lee states that in the context of financial crime compliance, “We can trust AI as much as any other model, as long as we anchor it with model risk management, data governance, oversight, and all the principles we currently have in place to oversee compliance models.”
Shining Light into the ‘Black Box’
When a user cannot access or understand an AI model's internal workings (algorithms), and decisions cannot be reasoned or explained, it is called a black box.
And if we cannot determine how AI has come up with its decisions, how can we trust the results?
As such, many banks and regulators are still reluctant to adopt new technologies due to a lack of explainability and operational issues. Yet, this explainability is more about human limitations in understanding complex information at scale.
Humans learn from a limited number of information tokens at a time. In contrast, these machines learn from hundreds of information tokens, parameters, and conditions and could, in theory, continue to grow infinitely. While humans can follow this path, we cannot understand it – equating these techniques to a black box.
“An algorithm being a black box should not be the sole determinant for using or not using a solution; there are ways to get around this. So, never dismiss the use of black box systems based on a lack of understanding” – Leandro Loss.
Advances in explainable AI and semi-standardized models, as well as methods such as post hoc explanation and independent vendor system model validation, are paving the way for faster adoption into the current regulatory system.
Listen to AML Voices: Model Validation – How to Handle Black Box Models here.
Why not simpler ‘white box’ models?
A white box model incorporates restraints that make the process more transparent. These models are the backbone of traditional rule-based compliance models.
However, bad actors spend as much time, if not more, innovating and learning new ways to bypass these anti-money laundering methods, and straightforward decision trees are easily circumvented.
Another challenge lies in the complex needs of most compliance regimes. A single entity could have hundreds and thousands of inputs, equating to even more words and syllable tokens. White box models are too basic and cannot keep up with the scale and complexity required for a successful compliance program.
“As a traditional rule-based system, we as an industry are nearing our ceiling, if not already at it.” – Kevin Lee.
Final thoughts
The need for AI models to detect anomalies and patterns of suspicious behavior should be a welcomed next step for banks and financial institutions to significantly improve their compliance function and realize additional efficiencies at scale.
But people will only use what they understand and can trust, and simply hitting the giant AI button isn’t going to get you any closer to your goal.
Institutions should actively look for specific use cases or opportunities to deploy AI in a way that they can control, understand, and measure against earlier benchmarks to advance next-gen modeling in this space.
Investment in the right talent, tools, and processes will support AI governance, ensuring comprehensible outcomes, mitigating risks, and fostering trust in the fairness and accuracy of the models' decisions.
Want to hear more? Listen to the podcast here!
Or, if you want to find out more about how we currently use the latest and finest technology to fight financial crime, fill out our contact form, and let’s start the conversation.